Adaptive Decision Making and Improved Data
Understanding for Experimental Science Using Statistical Machine Learning and
High Performance Computing
Jim
Ahrens
Los Alamos National Laboratory, Los Alamos,
NM, USA
Analyzing and extracting
scientific knowledge from modern science experiments has become the
rate-limiting step in the scientific process. We propose to accelerate knowledge-discovery from experimental
scientific facilities by combining high performance computing and statistical
science to produce an adaptive methodology and toolset that will analyze data and augment a scientist's decision-making so
that the scientist can optimize experiments in real time. We are developing
this capability in the context of dynamic compression experiments, an area of
core mission importance and an area that is currently in the midst of
substantial increases in the rate of data generation. This project will
result in a data science focused information science and technology toolset that
is optimized for and will revolutionize dynamic compression science
experiments using X-ray user facilities. Furthermore, this work will produce
many reusable components that can be applied to multiple scientific domains.
When achieved, our approach will allow scientists to elevate their focus
above the mundane tasks required for experiment completion to that of making
strategic scientific decisions.
Back to Session V
|
Quassical Computing
Ned
Allen
Lockheed – Martin Corporation, USA
We present a class of hybrid classical systems using
quantum co-processors and point out that unlike purely quantum computers,
such hybrids can be both universal and Turing complete; we introduce such
quantum-classical hybrids as “quassical.” We
discuss the benefits of quassical architectures
from a theoretical point of view: for some classes of problems they achieve
computational supremacy. From a practical point of view, quassical
architectures can also reduce the overhead burden imposed by most error
correction schemes and minimize the challenges of interconnecting qubits in a
usefully large connection graph. All quantum computing systems are
cyber-physical machines and thus quassical to at
least a trivial degree but only the more profoundly quassical
hybrids can exhibit an optimum problem-solving capability for the amount of
quantum resources deployed. Most significantly, quassical
architectures advance our thinking past that of seeing quantum machines as
simply quantum embodiments of classical ones and can enliven whole new fields
of analytical thinking that takes us beyond quantum information science per
se into a deeper understanding of the duality between quantum information and
fundamental thermodynamics, possibly suggesting unexpectedly useful new
technologies.
Back to Session VII
|
The Future is Collaborative: Paving the Way
for a Collaborative Computational Data Science Ecosystem for Big Data and Big
Compute
Ilkay Altintas
San Diego Supercomputer Center
and Computer Science and Engineering, Department University of California at
San Diego, USA
Our lives as well as any field of business and
society are continuously transformed by our ability to collect meaningful
data in a systematic fashion and turn that into value. These need not only
push for new and innovative capabilities in composable
data management and analytical methods that can scale in an anytime anywhere
fashion, anywhere, but also require methods to bridge the gap between
applications and such capabilities. However, we often lack collaborative
culture, effective methodologies and truly scalable collaborative tools to
translate these newest advances into impactful solution architectures that
can transform science, society and education.
FUTURE: A Collaborative Networked World as a Part of
the Data Science Process: Any solution architecture for data science today
depends on the effectivity of a multi-disciplinary data science team, not only
with humans but also with analytical systems and infrastructure which are
inter-related parts of the solution. Focusing on collaboration and
communication between people, and dynamic, predictable and programmable
interfaces to systems and scalable infrastructure from the beginning of any
activity is critical. This talk will overview some of our recent work on
dynamic data driven cyberinfrastructure and application solution
architectures. It will also introduce the family of composable
PPODS tools for team-based data science process management, explaining how
focusing on (1) some P’s in the planning phases of a data science activity
and (2) creating a measurable process that spans multiple perspectives and
success metrics will be effective in making computational data science
efforts scalable from the beginning.
Back to Session VIII
|
The Human Brain Atlas – why do we need
supercomputers?
Katrin
Amunts
Human Brain Project,
Chair of The Science and Infrastructure Board / Scientific Research Director,
Institute for Neuroscience and Medicine, Structural and Functional
Organisation of the Brain, Forschungszentrum Juelich GmbH, Juelich, Germany
and
Institute for Brain Research, Heinrich Heine
University Duesseldorf, University Hospital Duesseldorf, Germany
The human brain is a highly complex system, with
different levels of spatial organisation. E.g., on a macroscopic level, the
brain shows a highly variable folding pattern, while nerve cells on a microscopical level are arranged in layers and columns in
a regionally specific way. To capture the cellular architecture and study the
role of a specific brain region to function or behaviour requires to analyse
the brain in 3D. Deep-learning offers new tools to 3D reconstruct images of
histological sections at the microscopical scale,
and convolutional neuronal networks support to automatize brain mapping.
Considering the size of the brain with its nearly 86 billion nerve cells,
HPC-based workflows play an increasing role for developing high-resolution
brain models, to tame brain complexity.
Back to Session X
|
Pete Beckman
Exascale Technology
and Computing Institute, Argonne National Laboratory, Argonne, IL, USA
|
Quantum Computing at NASA
Rupak Biswas
Exploration Technology Directorate, High End
Computing Capability Project
NASA Ames Research Center,
USA
The success of many NASA missions depends on solving
complex computing challenges, some of which are NP-hard and intractable on
traditional supercomputers. Quantum computing promises an unprecedented
ability to solve intractable problems by harnessing quantum mechanical
effects such as tunneling, superposition, and
entanglement. The Quantum Artificial Intelligence Laboratory (QuAIL) at NASA Ames Research Center
is the space agency’s primary facility for conducting research and
development in quantum information sciences. The QuAIL
team conducts fundamental research in quantum physics but also explores how
best to exploit and apply this disruptive technology to enable NASA missions
in aeronautics, Earth and space sciences, and space exploration. In this
talk, I will give a brief overview of our efforts in quantum computing,
present recent results from some NASA application areas, and discuss challenges
and opportunities.
Back to Session VII
|
InfiniBand In-Network Computing Technology
and Roadmap
Gil
Bloch
HPC and Artificial Intelligence Arch, Mellanox Technologies, Sunnyvale, CA, USA
The latest revolution in HPC is the move to a
co-design architecture, a collaborative effort among industry, academia, and
manufacturers to reach Exascale performance by
taking a holistic system-level approach to fundamental performance
improvements. Co-design architecture exploits system efficiency and optimizes
performance by creating synergies between the hardware and the software.
Co-design recognizes that the CPU has reached the
limits of its scalability, and offers an intelligent network as the new “co-processor”
to share the responsibility for handling and accelerating application
workloads. By placing data-related algorithms on an intelligent network, we
can dramatically improve the data center and
applications performance.
Back to Session II
|
HPC in the Cloud - and update from the field
Brendan Bouffler
Scientific Computing Amazon Web Services,
London, USA
Software
and systems built in the public cloud have a tendency to innovate extremely
quickly. Last year, in 2017, Amazon Web Services (AWS) deployed almost 1500
new features and products on our platform alone. Our customers (a great many
of which are HPC users and HPC builders) of course leveraged these to create
even more new systems and services for their communities. It’s worth taking stock of the many
innovations that are available and distill a few
that are most prominent for HPC practitioners as well as the wider research
community who are just starting to leverage machine learning in their
environments. We’ll review some of the more impactful developments and
indicate where we think the next milestones will be marked in the many
journeys to the cloud.
Back to Session IX
|
Fogbow: a Middleware for the Federation of
IaaS Cloud Providers
Francisco
Brasileiro
Distributed Systems Lab, System and Computing
Department, Federal University of Campina Grande, Campina Grande, Brazil
The federation of Infrastructure-as-a-Service (IaaS)
cloud providers has been proposed as a way to improve their efficiency,
allowing them
not only to better accommodate the natural
fluctuations over time of their demands, but also to deal with users that
require their
applications to be deployed in a geographically distributed
fashion. In this talk we present the design and implementation of a
middleware that allows the fast and non-intrusive deployment of very large
federations of IaaS cloud providers. The use of the middleware in production
systems is also discussed, providing concrete evidences of its suitability.
Back to Session IX
|
Challenges and Opportunities for HPC
Interconnects
Ronald
Brightwell
Center for Computing Research, Sandia National Laboratories, Albuquerque,
NM, USA
This talk
will reflect on prior analysis of the challenges facing high-performance
interconnect technologies intended to support extreme-scale scientific
computing systems, how some of these challenges have been addressed, and what
new challenges lay ahead. Many of these challenges can be attributed to the
complexity created by hardware diversity, which has a direct impact on
interconnect technology, but new challenges are also arising indirectly as
reactions to other aspects of high-performance computing, such as alternative
parallel programming models and more complex system usage models. We will
describe some near-term research on proposed extensions to MPI to better
support massive multithreading and implementation optimizations aimed at
reducing the overhead of MPI tag matching. We will also briefly describe a
new portable programming model to offload simple packet processing functions
to a network interface that is based on the current Portals data movement
layer. We believe this capability will offer significant performance
improvements to applications and services relevant to high-performance
computing as well as data analytics.
Back to Session IV
|
Quantum Processing Units: A Post-Exascale Accelerator?
Jonathan
Carter
Computing Sciences Area, Computational
Research Division, Lawrence Berkeley National Laboratory, Berkeley, CA, USA
Tremendous progress has been made in the development
of quantum computing hardware over the past decade across many different experimental
platforms, including trapped neutral atom and ion systems, donor spins
embedded in semiconductors, and superconducting electrical circuits.
Semiconductor systems can leverage extremely high purity solid-state
materials and sophisticated materials processing techniques, but basic
scientific advancements are needed to realize large numbers of controllable
qubits with couplings suitable for logical gate operation. On the other hand,
both trapped ion and superconducting platforms are now in the position to
execute proof-of-concept quantum algorithms, though both approaches are far
from realizing universal computation with fault tolerant hardware.
At the same time, algorithms that can be
successfully executed on near-term noisy quantum hardware have been developed
or existing algorithms reformulated to reduce circuit-depth requirements - we
are entering an era of co-design for quantum computing. Many of these
algorithms are specialized to chemistry and materials science simulations,
where there has been rapid progress. I will cover the current developments in
this area and make some predictions as to whether we will see quantum
processing elements as a component of HPC systems emerge post-Exascale.
Back to Session VI
|
Data Compression For Quantum Population
Coding
Giulio Chiribella
Department of Computer Science, University of
Oxford, Oxford , UK
and
Department of Computer Science, The
University of Hong Kong,
Hong Kong, CHINA
Quantum states provide information about multiple,
mutually complementary observables. Such information is not accessible from a
single system, but becomes accessible when a population of many identically
prepared systems is available. In this context, an important question is how
much information is contained into n copies of the same state. A rigorous way
to quantify such information is through the task of quantum data compression,
where the goal is to store the quantum state into the smallest number of
quantum bits. The problem of compressing identically prepared systems is
relevant in several areas, including the design of quantum sensors that
collect data and transfer them to a central location, and the design of
quantum learning machines that store patterns in their internal memory. In
this talk I will characterize the minimum amount of memory needed to
faithfully store sequences of identically prepared quantum states, showing
how the size of the memory grows with the number of particles in the
sequence. In addition, I will discuss how much quantum memory can be traded
with classical memory. Finally, I will conclude by showing an application of
quantum compression to high precision measurements of time and frequency.
References for this talk:
Yuxiang Yang, Ge Bai, Giulio Chiribella, and Masahito Hayashi, Data compression for
quantum population coding, IEEE Transactions on Information Theory (2018),
10.1109/TIT.2017.2788407
Yuxiang Yang, Giulio Chiribella, and Masahito Hayashi, Optimal compression for
identically prepared qubit states, Physical Review Letters 117.9 (2016):
090502.
Yuxiang Yang, Giulio Chiribella, and Daniel Ebler.
Efficient quantum compression for ensembles of identically prepared mixed
states, Physical Review Letters 116.8 (2016): 080501.
Back to Session VI
|
Accelerating Materials Design and Discovery
with Data Science and Machine Learning
Alok Choudhary
Henry & Isabelle Dever
Professor of EECS, McCormick School of Engineering, EECS Department and
Kellogg School of Management, Northwestern University,
Evanston, IL, USA
Modern instruments, supercomputing simulations,
experiments, sensors and IoT are creating massive
amounts of data at an astonishing speed and diversity. This has the potential
to transform speed of discovery, thereby accelerating the pace of innovation
in materials, medicine to marketing and many disciplines in between. This
talk will present acceleration of materials design and discovery using data
science and machine learning.
Biography:
Alok Choudhary is the
Henry & Isabelle Dever Professor of Electrical
Engineering and Computer Science and a professor at Kellogg School of
Management. He is also the founder, chairman and chief scientist (served as
its CEO during 2011-2013) of 4C insights (formerly Voxsup
Inc.), a big data analytics and marketing technology software company. He
received the National Science Foundation's Young Investigator Award in 1993.
He is a fellow of IEEE, ACM and AAAS. His research interests are in
high-performance computing, data intensive computing, scalable data mining,
high-performance I/O systems, software and their applications in science,
medicine and business. Alok Choudhary
has published more than 400 papers in various journals and conferences and
has graduated 40+ PhD students.. Alok Choudhary’s work and interviews have appeared in many
traditional media including New York Times, Chicago Tribune, The Telegraph,
ABC, PBS, NPR, AdExchange, Business Daily and many
international media outlets all over the world.
Back to Session IX
|
High Performance Computing and Big Data:
Challenges for the Future
Jack Dongarra
Innovative Computing Laboratory, Computer
Science Dept.
University of Tennessee, Knoxville, TN
USA
Historically, high-performance computing advances
have been largely dependent on concurrent advances in algorithms, software,
architecture, and hardware that enable higher levels of floating-point
performance for computational models. Advances today are also shaped by
data-analysis pipelines, data architectures, and machine learning tools that
manage large volumes of scientific and engineering data.
We will examine some of the challenges involved with
high performance computing and big data for scientific computing.
Back to Session I
|
The Evolution of the EOSC in the Context of
the EOSC-Hub Project
Giacinto Donvito
INFN - Istituto Nazionale di Fisica Nucleare, EOSC – Hub Technology, Bari, ITALY
In the talk will be described the activities on
going and the roadmap for the evolution of the service catalogue that will
provide European researchers with a rich and powerful set of services in
order to exploit the available Cloud Resources for their scientific
activities. The talk will highlight the role of the EOSC-Hub project in the
context of the European Open Science Cloud initiative and how the foreseen
activities in the projects matches the overall movement in the European
context. A specific focus will be dedicate on how the scientific communities
are driving and contributing to this process.
Back to Session IX
|
The Upcoming Storm: The Implications of
Increasing Core Count on Scalable System Software
Matthew
Dosanjh
Center for Computing Research, SANDIA National Laboratories,
Albuquerque, NM, USA
As clock speeds have stagnated, the number of cores
has been drastically increased to improve processor throughput. Most scalable
system software has been developed for single-threaded environments.
Multi-threaded environments have seen a large uptake as application
developers leverage the full performance of the processor; however, these
environments are incompatible with a number of assumptions that have driven
scalable system software development. This presentation will highlight a case
study of this mismatch's impact on MPI message matching. MPI message matching
has been designed and optimized for traditional serial execution. The reduced
determinism in the order of MPI calls can significantly reduce the
performance of MPI message matching, potentially overtaking
time-per-iteration targets of many applications. Different proposed
techniques attempt to address these issues and enable multithreaded MPI
usage. These approaches highlight a number of tradeoffs
that make adapting MPI message matching complex. This case study and its
proposed solutions highlight a number of general concepts that need to be
leveraged in the design of next generation scaleable
system software.
Back to Session III
|
Extreme Scale Data Analysis and Machine
Learning for Science
Sudip S.
Dosanjh
National Energy Research Scientific Computing
Center
Lawrence Berkeley National Laboratory,
Berkeley, CA, USA
Scientific
data is exploding due to improvements in sensors, detectors and sequencers.
Large scale experimental instruments and observational facilities are
projected to generate Terabytes of data per second in the coming decade. In
environmental applications, the number of sensors is also increasing
dramatically. Gaining scientific insight from these large data sets requires
computing at an unprecedented level, as well as new algorithms that scale to
very high concurrency. This talk summarizes work at the National Energy
Research Scientific Computing (NERSC) Center to tackle
these big data challenges, as well as plans to create a Superfacility
for Science that ties together HPC centers and
experimental and observational facilities through high speed networks and
advanced software.
Back to Session VIII
|
System architecture opens up thanks to next
generation optics
Nicolas Dube
Exascale Systems Technology, HPe,
USA
It will focus on next generation system architecture
that goes beyond exascale or exaflops
and how co-packaged optics will change the economics, signal integrity and
energy efficiency of next generation supercomputers.
Back to Session II
|
Learning Systems for Science
Ian
Foster
Math & Computer Science Div., Argonne
National Laboratory
& Dept of
Computer Science, The University of Chicago, Chicago, IL, USA
New
learning technologies seem likely to transform much of science, as they are
already doing for many areas of industry and society. We can expect these
technologies to be used, for example, to obtain new insights from massive
scientific data and to automate research processes. However, success in such endeavors will require new learning systems: scientific
computing platforms, methods, and software that enable the large-scale
application of learning technologies. These systems will need to enable
learning from extremely large quantities of data; the management of large and
complex data, models, and workflows; and the delivery of learning
capabilities to many thousands of scientists. In this talk, I review these
challenges and opportunities and describe systems that my colleagues and I
are developing to enable the application of learning throughout the research
process, from data acquisition to analysis.
Back to Session I
|
High-Performance Big Data Computing
Environments
Geoffrey
Fox
School of Informatics, Computing and
Engineering, Department of Intelligent Systems Engineering, and Digital
Science Center and Data Science program
University of Indiana Bloomington, IN, USA
We analyse the components that are needed in
programming environments for Big Data Analysis Systems with scalable HPC
performance and the functionality of ABDS – the Apache Big Data Software
Stack. This motivates Twister2 which consists of a set of middleware
components to support batch or streaming data capabilities familiar from
Apache Hadoop, Spark, Heron and Flink but with high
performance
Twister2 covers bulk synchronous and data flow
communication; task management as in Mesos, Yarn
and Kubernetes; dataflow graph execution models; launching of the Harp-DAAL
library; streaming and repository data access interfaces, in-memory databases
and fault tolerance at dataflow nodes.
Similar capabilities are available in current Apache
systems but as integrated packages which do not allow needed customization
for different application scenarios.
Back to Session I
|
Moving Towards Personalized Medicine -
Simulating the Living Heart and the Living Brain with Cloud HPC
Wolfgang
Gentzsch
The UberCloud,
Germany
In the
last six years UberCloud has performed 200+ cloud
experiments with engineers and scientists and their complex applications.
Among others, recently, in a series of challenging high performance computing
applications in the Life Sciences, UberCloud’s HPC
Containers have been packaged with several scientific workflows and
application data to simulate complex phenomena in human’s heart and brain. As
the core software for these HPC Cloud experiments we used the (containerized)
Abaqus FEA solver running in a fully automated
multi-node multi-container HPE environment in the Advania
HPC Cloud. In this talk we present two
grand-challenge applications: Studying Drug-induced Arrhythmias of a Living
Human Heart with Abaqus 2017 in the Cloud
(Experiment 197); and Cloud Simulation of Neuromodulation in Schizophrenia
(Experiment 200).
Back to Session X
|
Vladimir Getov
Department of Engineering,
Faculty of Science and Technology
University of
Westminster, London, UNITED KINGDOM
|
A Systematic Approach to Developing
High-Performance, Portable GPU Programs
Sergei
Gorlatch
Universitaet Muenster, Institut
für Informatik, Muenster,
Germany
We
advocate the use of well-defined patterns and transformations for programming
modern many-core processors like Graphics Processing Units (GPU), as an
alternative to the currently used low-level, ad hoc programming approaches
like CUDA or OpenCL. Our new contribution is introducing an intermediate
level of low-level patterns in order to bridge the abstraction gap between
the popular high-level patterns and the executable code for many-cores. We
define our low-level patterns lbased on the OpenCL
programming model, and we introduce semantics-preserving rewrite rules that
transform programs with high-level patterns into programs with low-level
patterns, from which executable OpenCL programs are generated automatically.
We show that program design decisions and optimizations, which are usually
applied ad-hoc by experts, can be systematically expressed in our approach as
provably-correct transformations for high- and low-level patterns. We briefly
describe the current transformation-based system LIFT being developed under
the lead of the University of Edinburgh, which demonstrate how
automatically-generated OpenCL implementations for different application
areas that achieve performance competitive with programs that are manually
written and highly tuned by performance experts.
Back to Session III
|
Power of Analog Quantum Computers: Theory and
Reality
Itay Hen
University of Southern California,
Information Sciences Institute
Los Angeles, CA, USA
With recent breakthroughs in quantum technology,
large-scale analog machines that utilize the laws
of Quantum Mechanics to solve certain types of problems of practical
relevance are already becoming commercially available.
I will discuss recent developments in the field of analog quantum computing as well as our current
understanding of the power and limitations of analog
quantum computers.
Back to Session VI
|
HPC platform efficiency and challenges for a
system builder
Martin Hilgeman
High Performance Computing, DELL EMC,
Amsterdam, THE NETHERLANDS
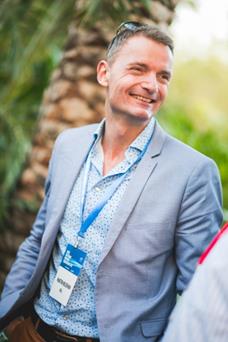
Martin Hilgeman (1973, Woerden, The Netherlands)
has a Master's Degree in Physical and Organic Chemistry obtained at the VU
University of Amsterdam. He has worked at SGI and IBM for 14 years as a
consultant, architect and as a member of the technical staff in the SGI
applications engineering group, where his main involvement was in porting,
optimization and parallelization of HPC applications.
Martin joined
Dell EMC in 2011, where he is acting as a Technical Director for HPC in
Europe, Middle East and Africa. His main interests are into application
optimization, modernization of parallel workloads and platform efficiency.
Lately, Martin has also accepted the responsibility for leading the
Artificial Intelligence strategy for Dell EMC in the region mentioned above.
Abstract
With all the advances in massively parallel and
multi-core computing with CPUs and accelerators, it is often overlooked
whether the computational work is being done in an efficient manner. This
efficiency is largely being determined at the application level and therefore
puts the responsibility of sustaining a certain performance trajectory into
the hands of the user. It is observed that the adoption rate of new hardware
capabilities is decreasing and lead to a feeling of diminishing returns. At
the same time, the well-known laws of parallel performance are limiting the
perspective of a system builder. The presentation tries gives an overview of
these challenges and what can be done to overcome them.
Back to Session II
|
Systems Packaging Technology for Efficient
Cooling for Dense HPC Solutions in a Data Center
Vinod
Kamath
LENOVO, Data Center
Group, Morrisville, North Carolina, USA
The computing architecture over the span of the past
decade has rapidly provided increases in rack performance with a steady
increase processor power. While the rate of growth in system performance was
non-linear the accompanying rack power consumption grew from about 20kW to
about 30kW for racks in the industry standard 19” footprint over the decade
using the industry standard X86 architecture. The rate of performance growth
needs to be maintained to deliver customer performance objectives, however
the processor and system power consumption trends are accelerating rapidly.
In the near term rack power consumption values in the 40-50 kW will be more
commonplace when packaged with the same processor socket density as prior
years. Traditional packaging technologies that use efficient air cooled
designs with enhanced efficient heatsinks, cooling fan power and system
airflow optimization are approaching limits of efficiency. Rapid increases in
all components that comprise a hpc system such as
processor, network, memory and NVMe disk power are
resulting in higher allocation of fan power to cool the system, and in some
instances a reduction in processor socket density in a rack to accommodate
the thermal design power of the CPU. Illustrative examples of a typical
compute node and rack with their power and cooling expectations will be
shown.
Lenovo has efficiency engineered into our system
designs that target improvements in cooling efficiency via heatsink optimization and fan power
optimization, examples of which will be shown. Datacenter
optimization has also required local
heat extraction at the rack. The engineering approach that describes
the traditional optimization will be described as one of the pillars of our
system design approach. Finally, as rack power values approach 40kW and are
trending to 1.5 times or higher from present values in the near future for
dense deployments, direct liquid to node cooling solutions are necessary.
Lenovo over the past 6 years has delivered HPC solutions with direct liquid
cooling at the node. Engineering to improve the cooling efficiency of such
solutions will be discussed. The TCO analysis that accompanies efficient liquid cooling solutions will be
presented with a method to evaluate the value of the deployment to the
customer.
Back to Session II
|
Non-Quantum Effects in Data Production
Carl Kesselman
Department of Industrial and Systems
Engineering, Information Sciences Institute, University of Southern
California
Marina del Rey, Los Angeles, CA, USA
It is unfortunately the case that many published scientific
results are unreproducible. Recent
studies have shown that results cannot be reproduced in as few as 1 out of 10
papers published in top tier journals.
While there are many factors that cause unreproducible results, bad
data practices definitely play an non-trivial contributing role with an
impact spanning many disciplines from computer science to biology. With the increased influence of big data
and cloud based scalable computing, this problem will only get worse. In spite of the scale of the problem, the
practicing scientist has few practical tools available to help create
reproducible data. To address this gap, we have developed some basic tools
and techniques that promote the creation of reusable scientific data on
diverse computational platforms, within the context of complex and evolving
scientific investigations. In my talk,
I will present some of these tools and describe how they are being used in
practice to enhance scientific reporducablitqy
across a broad array of scientific use cases.
Back to Session II
|
Hiroaki Kobayashi
Architecture
Laboratory, Department of Computer and Mathematical Sciences
Tohoku University,
Sendai Miyagi, JAPAN
|
Road towards exascale – comments on the practical and economical
aspects
Kimmo Koski
CSC - Tieteen tietotekniikan keskus (CSC - IT
Center for Science), Espoo, Finland
During the recent
years number of countries, computer vendors and research infrastructures have
introduced their plans for enabling Exascale-level
computing infrastructure. European initiative EuroHPC
plans to install of two pre-Exascale systems during
the next few years and two Exascale systems in
about 4-5 years. Estimated power envelopes vary between 10 – 50 MW,
capabilities which are not available in every location. Total cost of
ownership can be dominated by electricity cost, although new innovative datacenter technologies are being developed. Need for
balanced HPC ecosystem instead vs. just providing peak performance computing
power depends on the required applications.
Economical aspects
of providing Exascale are emerging – can anyone
afford to run such a system? Practical
considerations about what do we actually want to achieve with the capability
and how to make the complex environment work efficiently are sometimes
forgotten instead of looking for breaking news about being able to break the Exaflop/s barrier in LINPACK.
The talk introduces
the on-going Finnish data-intensive HPC procurement and the scientific case
justifying the investment decision. Six different areas of use cases are
presented – each of them with a need for exascale
computing. Requirements and cost models for future exascale
installations are discussed, including datacenter
operations and constructions. CSC Kajaani datacenter is used as a
case example of when discussing the benefits and challenges for running a datacenter targeting to exaflop.
Back to Session X
|
Cloud Federation as an Evolutionary Path from
Grid Computing
Craig
Lee
Computer Systems
Research Dept., The Aerospace Corporation, El Segundo, CA USA
The need
to manage flexible, on-demand collaborations is fundamental.
The grid computing
concept was motivated by the desire to support international "big
science" collaborations. Fast
forward fifteen years. We are now in
the cloud computing, big data, and IoT era. The need for flexible collaborations is
more acute than ever. Inherently
distributed collaboration environments can be called federations.
Such
federations must address all the same fundamental requirements as grids. Given the continued development of widely
adopted distributed computing tools, however, very different implementation approachs are possible.
In
response to the growing awareness of the need for standardized federation
capabilities, the National Institute of Standards and Technology and the IEEE
have established coordinated working groups to address cloud federation.
The real
work of this group is to engage all manner of stakeholders and to promote an
emerging best practice around federation that becomes self-sufficient.
Back to Session IX
|
Thomas Lippert
Juelich Supercomputing
Centre, Forschungszentrum Juelich
Juelich, GERMANY
|
Deploying
Complex User Applications over Hybrid Cloud Deployments Based on Open
Standards
Álvaro López García
Spanish National Research Council (CSIC),
Santander, Spain
The DEEP-Hybrid-DataCloud
project aims at delivering a feature rich platform as a service layer that
will provide easy access to cloud resources leveraging specialized hardware
(such as accelerators) in order to execute intensive applications for
scientific usage (like deep learning applications). In order to overcome the
limits both in scale and in capabilities that using a single private cloud
may impose, a high level hybrid cloud approach is used. This way, the
developed hybrid cloud platform will transparently (both for the users and the providers)
connect different IaaS services, being able to support the user workloads,
providing access to specialized hardware accelerators and data services that
span several resource providers. In this talk we will illustrate how the
DEEP-Hybrid-DataCloud is carrying out this approach
relying on the OASIS TOSCA open standard, in order to ensure proper
interoperability across different resource provider and cloud management
frameworks.
Back to Session IX
|
The EGI
Federated Cloud Status and Future Evolution
Álvaro López García
Spanish National Research Council (CSIC),
Santander, Spain
The European Grid Infrastructure has been building
out support for federated clouds for a number of years. This has included the integration of the
federation capabilities in the OpenStack Keystone service. This is partially
motivated by need to for more web-friendly tooling. This talk will present plans for future
evolution and the wider adoption of standardized approaches.
|
Towards Next Generation Chinese Supercomputer
Yutong Lu
National Supercomputing Center
in Guangzhou
School of Computer Science
National University of Defense
Technology
China
Supercomputing
technology has been developing very fast, impacted the science and society
deeply and broadly. Computing-driven and Bigdata-driven
scientific discovery has become a necessary research approach in global
environment, life science, nano-materials, high
energy physics and other fields. Furthermore, the rapidly increasing
computing requirements from economic and social development also call for the
power of Exascale system. Nowadays, the development
of computing science, data science and intelligent science has brought new
changes and challenges in system, technology and application of HPC. The
usage mode and delivery mode based on cloud computing also attracts
supercomputer users. The future Exascale system
design faces many challenges, such as architecture, system software,
application environment and so on. The report will analysis the usage mode of
the current Supercomputing Center, then discuss the
design and application environment of future super computing system.
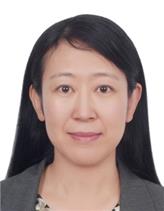
Bio:
Professor Yutong Lu is the Director of
National Supercomputing Center in Guangzhou, China.
She is the professor in School of Computer Science, Sun Yat-sen
University as well as in National University of Defense
Technology (NUDT). She is a member of Chinese national key R&D plan HPC
special expert committee She got her B.S, M.S, and PhD degrees from the NUDT.
Her extensive research and development experience has spanned several
generations of domestic supercomputers in China. Prof.
Lu is deputy chief designer of Tianhe Project. She
had won first class award and outstanding award of Chinese national science
and technology progress in 2009 and 2014 respectively. She is leading several
innovation projects on HPC and Bigdata supported by
MOST, NSFC and Guangdong Province now. Her continuing research interests
include parallel operating systems (OS), high-speed communication, large
scale file system& data management, advanced HPC/BD/AI convergent
application environment.
Back to Session IV
|
From Post-K to
Cambrian Explosion of Computing and Big Data in the
Post-Moore Era
Satoshi
Matsuoka
RIKEN Center for
Computational Science, Kobe and
Department of Mathematical and Computing
Sciences
Tokyo Institute of Technology, Tokyo, JAPAN
The so-called “Moore’s Law”, by which the
performance of the processors will increase exponentially by factor of 4
every 3 years or so, is slated to be ending in 10-15 year timeframe due to
the lithography of VLSIs reaching its limits around that time, and combined
with other physical factors. Based on the expected results from the Post-K
supercomputer at RIken CCS, we are also now
embarking on a project to revolutionize the total system architectural stack
in a holistic fashion in the Post-Moore era, from devices and hardware,
abstracted by system software and programming models and languages, and
optimized according to the device characteristics with new algorithms and
applications that exploit them. Such systems will have multitudes of
varieties according to the matching characteristics of applications to the
underlying architecture, leading to what can be metaphorically described as
Cambrian Explosion of computing systems. The diverse elements of such systems
will be interconnected with next-generation terabit optics and networks,
allowing metropolitan-scale computing infrastructure that would truly realize
high performance parallel and distributed computing.
However, which algorithms and applications would benefit
the most from such future computing, given that some physical constants,
e.g., communication latency, cannot be improved? We speculate on some of the
scenarios that would change the nature of current Cloud-centric
infrastructures towards the Post-Moore era.
Back to Session I
|
Simulation on and
HPC simulation of quantum computers and quantum annealers
Kristel Michielsen
Institute for Advanced Simulation, Quantum
Information Processing Group, Jülich Supercomputing
Centre, Forschungszentrum Jülich,
and RWTH Aachen University, Germany
A quantum computer (QC) is a device that performs
operations according to the rules of quantum theory. There are various types
of QCs of which nowadays the two most important ones considered for practical
realization are the gate-based QC and the quantum annealer
(QA). Practical realizations of gate-based QCs consist of less than 100
qubits while QAs with more than 2000 qubits are commercially available.
We present results of simulating on the IBM Quantum
Experience devices with 5 and 16 qubits and on the D-Wave 2X QA with more
than 1000 qubits. Simulations of both types of QCs are performed by first modeling them as quantum systems of interacting spin-1/2
particles and then emulating their dynamics by solving the time-dependent
Schrödinger equation. Our software allows for the simulation of a 48-qubit
gate-based universal QC on the Sunway TaihuLight
and K supercomputers.
References:
K. Michielsen, M. Nocon, D. Willsch, F. Jin, T. Lippert, H. De Raedt, Benchmarking gate-based quantum computers,
Comp. Phys. Comm. 220, 44 (2017)
D. Willsch, M. Nocon, F. Jin, H. De Raedt, K. Michielsen, Gate
error analysis in simulations of quantum computers with transmon
qubits, Phys. Rev. A 96, 062302 (2017)
H. De Raedt, F. Jin, D. Willsch, M. Nocon, N. Yoshioka, N. Ito, S. Yuan, K. Michielsen, Massively
parallel quantum computer simulator, eleven years later, arXiv:1805.04708
D. Willsch, M. Nocon, F. Jin, H. De Raedt, K. Michielsen, Testing quantum fault tolerance on small
systems, arXiv:1805.05227
K. Michielsen, F. Jin, and H. De Raedt, Solving 2-satisfiability problems on a
quantum annealer (in preparation)
Back to Session VI
|
MRG8:Random Number Generator for the
Million-plus core Era
Kenichi
Miura, Ph.D.
Fujitsu Laboratories of America and Lawrence
Berkeley National Laboratory
Sunnyvale, CA, USA
Pseudo random number generators (PRNGs) are crucial
for various simulations in HPC. These applications require high throughput
and good statistical quality from the PRNGs – especially for parallel
computing where long pseudo-random sequences can be exhausted rapidly. Although a handful PRNGs have been adapted
to parallel computing, they do not fully exploit the features of
wide-SIMD many-core processors and GPU
accelerators in modern supercomputers.
Multiple Recursive Generators (MRGs) are a family of
random number generators based on higher order polynomials, which provide
statistically high-quality random number sequences with extremely long
periods, and jump-ahead scheme for effective parallelization.
Since our talk in 2014, we reformulate the MRG8
(8th-order recursive implementation) for Intel’s KNL and NVIDIA’s P100 GPU –
named MRG8-AVX512 and MRG8-GPU respectively.
Our optimized implementation generates the same
random number sequence as the original well-characterized MRG8. We evaluated
MRG8-AVX512 and MRG8-GPU together with vender tuned random number generators
for Intel KNL and GPU. MRG8-AVX512 achieves a substantial 69% improvement
compared to Intel’s MKL, and MRG8-GPU shows a maximum 3.36x speedup compared
to NVIDIA’s cuRAND library.
This study has been conducted together with Mr.
Yusuke Nagasaka of Tokyo Institute of Technology
and Dr. John Shalf of Lawrece Berkeley Laboratory.
Back to Session X
|
Towards quantum-assisted optimization and
machine learning on Google Quantum Cloud
Masoud Mohseni
Quantum Artificial Intelligence Laboratory, Google
Inc., Venice, CA, USA
We present an overview of our progress on quantum
optimization and machine learning at Quantum AI Lab at Google. In particular,
we present an end-to-end quantum-assisted optimization engine on Google Cloud
Platform. Our physics-inspired approaches use an interplay of thermal and
quantum fluctuations to sample from unaccessible
low-energy states of spin-glass systems that encode certain hard
combinatorial optimization and probabilistic inference problems. We introduce
structured droplet instances and show that our hybrid quantum-classical
heuristic algorithms can significantly improve over classical techniques,
such parallel tempering, that rely on local updates. We also introduce
universal discriminative quantum neural networks for classification and
purification of quantum data. We train near-term small-scale quantum circuits
to classify data represented by non-orthogonal quantum probability
distributions using stochastic optimization techniques. This is achieved by
iterative interactions of a classical processor with a quantum device to
discover the parameters of an unknown non-unitary quantum map which can
implemented via a shallow quantum circuit.
Similar small-scale quantum circuit learning could be used for
verifying the quantum outputs of other shallow circuits, constructing
structured receivers in quantum imaging/sensing, and designing quantum
repeaters in quantum communication networks.
Back to Session VI
|
Achieving bit-wise reproducible results on
Anton, a special-purpose supercomputer for molecular dynamics simulation
Mark Moraes
Engineering Department, D. E. Shaw Research,
New York, N.Y., USA
The ability to exactly reproduce the output of
scientific simulations, often called bit-wise reproducibility (BWR), is
rarely achieved in parallel scientific software, especially across different
sizes of machines. Anton is a
massively parallel special-purpose machine that accelerates molecular
dynamics simulations by orders of magnitude compared with the previous state
of the art. Anton's algorithms,
hardware, and software were designed from the outset to achieve such
reproducibility, and this capability has been invaluable to the biochemistry
researchers who use Anton as well as the Anton engineering and operations
teams. For scientists, BWR allows
simulations to be extended as needed, and output size greatly reduced since
they can 'zoom' in to interesting parts of a simulation by re-running those
parts as needed. For engineers and the
operations staff, hardware bugs can be avoided during design verification
while software and algorithmic 'bugs' can be isolated quickly. I will discuss what it took to achieve
Anton's unique bit-wise reproducibility and show some examples of its value.
Back to Session II
|
Machine Learning on In-house HPC
Yuichi
Nakamura
Central
Research Laboratories, NEC,
Kanagawa, JAPAN
Lately, HPC is going to use for machine learning applications
in addition to large scale simulation. However, machine learning application
needs huge data sets and such huge data might include serious security and
privacy issues. Then, a concept of in-house HPC or inside HPC is introduced.
We think servers with GPGPU card is one of in-house HPC. Then, we NEC, released card base vector processors,
SX-Aurora Tsubasa as one of an accelerator board
for in-house HPC. In this talk, I would like to introduce some machine
learning use cases with SX-Aurora-Tsubasa as an
in-house HPC. Then, I will present a machine resource extension method to
in-house HPC when machine resources are in short.
Back to Session V
|
Scientific Workflows, Big Data, and
Extreme-Scales: Challenges, Opportunities and Some Solutions
Manish Parashar
Dept. of Computer Science, Rutgers
University, Piscataway, NJ, USA
Data-related
challenges are quickly dominating computational and data-enabled sciences and
are limiting the potential impact of scientific application workflows enabled
by current and emerging extreme scale, high-performance, distributed
computing environments. These data-intensive application workflows involve
dynamic coordination, interactions and data coupling between multiple
application processes that run at scale on different resources, and with
services for monitoring, analysis and visualization and archiving, and
present challenges due to increasing data volumes and complex data-coupling
patterns, system energy constraints, increasing failure rates, etc. In this
talk I will explore some of these challenges and investigate how solutions
based on data sharing abstractions, managed data pipelines, data-staging
service, and in-situ / in-transit data placement and processing can be used
to help address them. This research is part of the DataSpaces
project at the Rutgers Discovery Informatics Institute.
Back to Session VIII
|
Extreme Data Management Analysis and
Visualization
for Exascale
Supercomputers and Experimental Facilities
Valerio
Pascucci
University of Utah, Center
for Extreme Data Management, Analysis and Visualization, Scientific Computing
and Imaging Institute, School of Computing
and Pacific Northwest National Laboratory,
Salt Lake City, UT, USA
Effective use of data management techniques for
analysis and visualization of massive scientific data is a crucial ingredient
for the success of any supercomputing center and
cyberinfrastructure for data-intensive scientific investigation. In the
progress towards exascale computing, the data
movement challenges have fostered innovation leading to complex streaming
workflows that take advantage of any data processing opportunity arising
while the data is in motion.
In this talk I will present a number of techniques
developed at the Center for Extreme Data Management
Analysis and Visualization (CEDMAV) that allow to build a scalable data
movement infrastructure for fast I/O while organizing the data in a way that
makes it immediately accessible for analytics and visualization. In addition,
I will present an advanced in-situ data analytics framework that allows
processing data on parallel supercomputers without requiring advanced user
knowledge of parallel computing or advanced runtime systems.
Overall, this leads to a flexible data streaming
workflow that allows working with massive simulation models or data from high
resolution experimental facilities without compromising the interactive
nature of the exploratory process that is characteristic of the most effective
data analytics and visualization environment.
BIOGRAPHY
Valerio Pascucci is the Inaugural John R. Parks Endowed Chair of the
University of Utah and the founding Director of the Center
for Extreme Data Management Analysis and Visualization (CEDMAV) of the
University of Utah. Valerio is also a Faculty of the Scientific Computing and
Imaging Institute, a Professor of the School of Computing, University of
Utah, and a Laboratory Fellow, of PNNL and a visiting professor in KAUST.
Before joining the University of Utah, Valerio was the Data Analysis Group
Leader of the Center for Applied Scientific
Computing at Lawrence Livermore National Laboratory, and an Adjunct Professor
of Computer Science at the University of California Davis. Valerio's research
interests include Big Data management and analytics, progressive
multi-resolution techniques in scientific visualization, discrete topology,
geometric compression, computer graphics, computational geometry, geometric
programming, and solid modeling. Valerio is the coauthor of more than two hundred refereed journal and
conference papers and is an Associate Editor of the IEEE Transactions on
Visualization and Computer Graphics.
Back to Session VIII\
|
Supervised learning
on quantum computers
Francesco
Petruccione
Quantum Research Group, Quantum Information
Processing and Communication
School of Chemistry and Physics, University
of KwaZulu-Natal, Durban,
SOUTH AFRICA
Quantum Machine Learning is an emerging discipline, that
has attracted considerable interest recently. This is motivated, on the one
hand, by the obvious fact that artificial intelligence and machine learning
are central to the Fourth Industrial Revolution. On the other hand, noisy-intermediare
scale quantum (NISQ) computers, as well as quantum annealers,
are now available in the cloud. The talk gives an overview of the status of
quantum machine learning and explores the possibility of using NISQ Computers
for machine learning.
Back to Session VI
|
Acqua: Building Chemistry,
AI and Optimization Quantum Applications
Marco
Pistoia
Quantum Computing Software, IBM Watson Research Center, NY, USA
Problems that
can benefit from the power of quantum computing have been identified in numerous domains, such as Chemistry,
AI, Optimization and Finance. Quantum computing, however, requires very specialized skills. To address the needs of the vast population of practitioners who want to use and contribute to
quantum computing at various levels of the software stack, we have
created Acqua, a modular and extensible
library of quantum algorithms
that can be invoked directly or via domain-specific
applications. In this
talk, we motivate the need
for a quantum computing software stack, and present Acqua and its Chemistry, AI and Optimization applications.
Back to Session VI
|
High-Performance Big Data Computing with
Harp-DAAL
Judy Qiu
School of Informatics and Computing and Pervasive
Technology Institute, Indiana University, USA
Telemetry sensor’s data plays a major role in many
areas such as motor racing, meteorology, agriculture, transportation,
manufacturing processes and energy monitoring. In the domain of motor racing,
a car has over 50 of such sensors that generate a lot of data on logging
readings and presents a challenging big data problem. The importance of using
the fastest data processing technology in a sport is all about speed, from a
calculation of the next move based on information gathered during the race to
anomaly detection in streaming. To enable car simulators and analytics
on-the-fly for the Indianapolis 500 racing application, we leverage a novel
HPC-Cloud convergence framework named Harp-DAAL and demonstrate that the
combination of Big Data and HPC techniques can simultaneously achieve
productivity and performance. Harp is a distributed Hadoop-based framework
that orchestrates efficient node synchronization. Harp uses Intel® Data
Analytics Accelerator Library (DAAL), for its highly optimized kernels on
Intel® Xeon and Xeon Phi architectures. This way the high-level API of Big
Data tools can be combined with intra-node fine-grained parallelism, which is
optimized for HPC platforms for machine learning and complex data analytics.
We show how simulations and Big Data analytics can use common programming
environments with a runtime based on a rich set of collectives and libraries
of Harp-DAAL.
Back to Session VIII
|
Beyond Moore’s Law: Quantum Computing at Los
Alamos
Avadh Saxena
Los Alamos National Lab., USA
With classical computing reaching its theoretical
limits, new paradigms that go beyond Moore’s law have become imperative.
Quantum computing, neuromorphic computing and inexact (or probabilistic)
computing are three alternatives. I will mostly focus on significant recent
efforts devoted to quantum computing at Los Alamos. These involve both
gate-based quantum computing and using a quantum computer as an annealer for optimization problems. New quantum
algorithms and error correcting codes are being developed to address real
problems such as those involving linear solvers, sampling, graph
partitioning, efficient combinatorial optimization, many-body physics, quantum
chemistry, among others. Fundamental aspects, e.g. entanglement and decoherence, as well as quantum machine learning and
quantum control protocols will be discussed. Finally, I will delve into some
aspects of hardware (e.g. superconducting qubits vs trapped-ion qubits,
etc.).
Back to Session VII
|
Next-Generation Computing: Transitioning
Beyond-Silicon Technologies from Idea to Reality
Max Shulaker
Microsystems Technology Laboratories,
Department of Electrical Engineering and Computer Science, Massachusetts
Institute of Technology, Boston, MA, USA
At this exact moment when future applications are
demanding massive improvements in computing performance, conventional
approaches to improving computing are becoming increasingly challenging. For
instance, silicon CMOS scaling (Dennard scaling and equivalent scaling) has
already slowed due to the power wall.
Moreover, abundant-data applications are increasingly dominated by the time
and energy required to transfer data between computing engines (e.g.,
domain-specific accelerators, general-purpose processors) and off-chip memory
(the memory wall). It is clear that
business as usual is inadequate. To overcome these multiple walls (power
wall, memory wall) and enable the next leaps in computing system
capabilities, isolated improvements in logic or memory technologies alone are
insufficient. Rather, improved technologies such as beyond-silicon
nanotechnologies, in conjunction with new computing architectures that finely
integrate logic and memory, will enable the next leap demanded by the coming
generations of transformative abundant-data applications. For instance,
carbon nanotube (CNT)-based transistors promise an order of magnitude benefit
in energy efficiency versus silicon CMOS, while resistive RAM (RRAM) promises
massive on-chip non-volatile memory. Moreover, due to the unique low
temperature fabrication of transistors built using CNTs and memories from
RRAM, these two emerging technologies together enable monolithic 3D integrated
circuits - whereby layers of logic and
memory are fabricated directly vertically over one-another, interleaving
logic and memory within a three-dimensional stack. In this talk, I will
describe major advancements towards realizing such future systems, and
describe how significant efforts underway could shape the next-generation of
computing systems.
Back to Session III
|
Contemplating Non-von Neumann Computing for Zetaflops and Dynamic Graphs
Thomas
Sterling, Maciej Brodowicz,
Matthew Anderson
Department of Intelligent Systems
Engineering, School of Informatics, Computing, and Engineering, Indiana
University, USA
At the
risk of stating the obvious, HPC is entering a point of singularity where
previous technology trends (Moore’s Law etc.) are terminating and dramatic
performance progress may depend on advances in computer architecture outside
of the scope of conventional practices. This may extend to the opportunities
potentially available through the context of non-von Neumann architectures.
Curiously, this is not a new field but suffered from the relatively easy
growth potential powered by decades of Moore’s Law including resulting
improvements in device density and clock rates. Cellular automata, static and
dynamic data flow, systolic arrays, and neural nets have demonstrated
alternative approaches to von Neumann derivative architectures throughout
past decades, each exhibiting unique advantages but also imposing open
challenges and time to delivery. A new class of non von Neumann architecture,
the Simultac, is being pursued and recent scaling
studies suggest that its genus or structures, called here “Continuum Computer
Architecture (CCA)” of which the Simultac is just
one, has the possibility to scale many orders of magnitude beyond present day
HPC systems. Further, by incorporating select mechanisms for the purpose, it
may greatly enhance dynamic graph processing even further. This presentation
will describe elements of this study on the scaling of CCA and suggest with a
change in enabling technology towards the latter half of the next decade may
yield at least peak capabilities of Zetaflops and
beyond at practical power, size, and cost. Questions from participants are
welcome throughout the presentation.
Back to Session I
|
Computing Landscape 2030: New Architectures and Computing Models,
Machine Learning Based Software, Neurons and
Entanglement
Rick
Stevens
Argonne National Laboratory and Department of
Computer Science, The University of Chicago, Argonne and Chicago, USA
Earlier this year I generated a series of fanciful
future scenarios for computing that posited an aggressive, and somewhat
chaotic synthesis of trends, in this talk I’ll dive deeper and try to put
some analysis behind these trends and directions. In these scenarios –
instantiated every five years for the next fifteen – I try to weave together
what our computing environments might become.
During this time Moore’s law drives to 4nm and then perhaps one or two
more turns. Innovation in architecture
(and circuit optimization) becomes the dominate (perhaps only) source of
increased performance in classical computing.
Software moves from hand-crafted works-of-art to machine optimized
mashups of mostly machine generate codes derived from some data, both natural
data from the world and data generated by previous generations of hand-built
software. Hardware design also will be
influenced by machine learning based optimization tools, but increasingly
will be targeted at machine learning dominated workloads.
A key challenge for the AI push of the next decade
will be the smooth integration of all the theoretical knowledge we have
accumulated at great cost with the data driven learned representations of the
world. The quest for ever
increasingly energy efficient circuits and systems will push towards very
non-Von type computing structures, spreading computing elements throughout
the machine, into memories, interconnects, storage systems, etc. Extreme versions of novel computing designs
will build on ideas from neuroscience and neuromorphic computing among
others. For some problems, perhaps
large classes of data driven problems, neuromorphic designs might emerge as
peer computing platforms with classical devices. For other problems they might be viewed as
hardware instantiations of simulators for neuroscience. Lurking in the corner is quantum. Quantum based computing might breakout
before 2030 beyond its use as a curiosity cabinet and perhaps somewhat more
useful use as an analog simulator for quantum
phenomena. One of the more intriguing
possible uses of quantum computing is for machine learning where the system
can learn quickly on superimposed training data. This use case for quantum computing puts
enormous pressure on the development of quantum memories and quantum sensing,
where the data might come pre-superimposed.
How all of these forces and more might or might not come together is
the topic of this talk.
Back to Session I
|
Multi-scale simulation of Ras
proteins on lipid bilayers
Frederick H. Streitz,
Lawrence Livermore National Laboratory
Frederick
Streitz
High Performance Computing Innovation Center, Lawrence Livermore National Laboratory,
Livermore, CA, USA
Simulating proteins on lipid membranes could provide
unprecedented insights into cancer biology and a host of other phenomena.
However, such simulations face conflicting and seemingly insurmountable
constraints: reaching biologically relevant time and length scales (milliseconds
and microns) requires continuum-level models but understanding the processes
of interest requires molecular level detail. I will present a new type of
massively parallel, multi-scale simulation framework that brings together
these two modeling paradigms. Using
state-of-the-art machine learning, we couple a novel continuum model to an
ensemble of molecular dynamics simulations. By carefully selecting MD
simulations we ensure that the entire phase space explored by the continuum
model is adequately sampled and explored at the finer scale. The result is a
simulation at macro length- and time-scales that incorporates micro-scale
precision.
Back to Session X
|
Sometimes the complexity really IS
exponential
Francis
Sullivan1
IDA/Center for
Computing Sciences, Bowie, MD, USA
Problems
whose solutions require exascale capabilities can
be characterized, in part, by their size, as measured by amounts of data
produced, accessed, and moved. But equally important is their computational
complexity, mean- ing the amount of computation
required, f (n) where n measures the size of the instance. In a perfect
world, the function f is a polynomial and some problem instances parallelize.
(Think matrix inversion.) But in the world in which we live, we encounter f
(n) = O(2n) and the problem resists all efforts to parallelize. (Think
3-SAT.) In these cases, we can try to put a lot of thought into algorithm
design, in the hope of reducing O(2n) to O((1 + η)n) where η
<< 1. Sometimes this can be accomplished by bringing novel math- ematical tools to bear on the question.
We
illustrate this approach by describing a method for approximating all of the coefficients of the all terminal
reliability problem. Our method makes use of standard computational tools
such as low-rank updates but it also makes use of combinatorial techniques
not usually associated with numerical
computation.
1. Joint work with David G. Harris
Back to Session VIII
|
Digital Annealer:
Quantum-inspired Computing for Combinatorial Optimization Problems
Kazuya
Takemoto
Technology Development Group, Digital Annealer Project, Fujitsu Laboratories Ltd., Kawasaki,
JAPAN
Fujitsu digital annealer
(DA) is a newly-developed computing architecture dedicated for hard-to-solve
combinatorial optimization problem. So far, quantum annealing has been widely
studied as a metaheuristic method for solving such combinatorial optimization
problems. However, current quantum annealing processor has technical
limitations such as a sparse connectivity between qubits and discrete
weights. This may cause significant overhead cost when applying to
complicated industrial problems.
Digital annealer is a digital-circuit-based
accelerator for Markov chain Monte Carlo stochastic search. It is designed to
handle 1,024-bit Ising spins, which are fully
connected through 16-bit weights. We have implemented two accelerating
techniques: one is a parallel trial scheme, and the other is a transition
facilitation technology. These features facilitate to solve practical
large-scale combinatorial optimization problems using DA.
In this talk we will describe the architecture
design and future prospects of DA. Several demonstrations for chemical,
medical and financial applications are also presented.
Back to Session VII
|
Domenico Talia
Department of
Computer Engineering, Electronics, and Systems and DtoK Lab
University of
Calabria, ITALY
|
Deep Learning Acceleration of Progress toward
Delivery of Fusion Energy
William
Tang
Princeton University, Dept. of Astrophysical
Sciences, Plasma Physics Section, Princeton Plasma Physics Laboratory, and Princeton
Institute for Computational Science and Engineering, Princeton, USA
Accelerated
progress in producing accurate predictions in science and industry have been
accomplished by engaging modern big-data-driven statistical methods featuring
machine/deep learning/artificial intelligence (ML/DL/AI). Associated
techniques being formulated and adapted have enabled new avenues of
data-driven discovery in key scientific applications areas such as the quest
to deliver Fusion Energy – identified by the 2015 CNN “Moonshots
for the 21st Century” series as one of 5 prominent grand challenges. An
especially time-urgent and very challenging problem facing the development of
a fusion energy reactor is the need to reliably predict and avoid large-scale
major disruptions in magnetically-confined tokamak systems such as the
EUROFUSION Joint European Torus (JET) today and the burning plasma ITER
device in the near future. Significantly improved methods of prediction with
better than 95% predictive accuracy are required to provide sufficient
advanced warning for disruption avoidance or mitigation strategies to be
effectively applied before critical damage can be done to ITER -- a
ground-breaking $25B international burning plasma experiment with the
potential capability to exceed “breakeven” fusion power by a factor of 10 or
more. This truly formidable task demands accuracy beyond the near-term reach
of hypothesis-driven /”first-principles” extreme-scale computing (HPC)
simulations that dominate current research and development in the field.
Recent
HPC-relevant advances in the deployment of deep learning recurrent and
convolutional neural nets in Princeton’s new Deep Learning Code -- "FRNN
(Fusion Recurrent Neural Net) Code on modern GPU systems. This is clearly a
“big-data” project in that it has direct access to the huge JET disruption
data base of over a half-petabyte to drive these studies. FRNN implements a
distributed data parallel synchronous stochastic gradient approach with Tensorflow libraries at the backend and MPI for
communication. This deep learning software has demonstrated excellent scaling
up to 6000 GPU's on “Titan” at the Oak Ridge National Laboratory – an
achievement that has helped establish the practical feasibility of using
leadership class supercomputers to greatly enhance training of neural nets to
enable transformational impact on key discovery science application domains
such as Fusion Energy Science.
Powerful
systems on which FRNN is currently deployed include: (1) Japan’s TSUBAME 3 –
where over 1000 Pascal P100 GPU's have already enabled impressive
hyper-parameter tuning production runs; and (2) ORNL’s SUMMIT featuring the
new VOLTA GPU’s on which FRNN’s new “half-precision” algorithmic capability
has produced attractive scaling results. Summarily, statistical Deep Learning
software trained on very large data sets hold exciting promise for delivering
much-needed predictive tools capable of accelerating scientific knowledge
discovery in HPC. The associated creative methods being developed also has
significant potential for cross-cutting benefit to a number of important
application areas in science and industry.
Back to Session V
|
Modeling the Next-Generation High Performance
Schedulers
Michela Taufer
Dept. of Computer and Information Sciences,
Biomedical Engineering
and Center for
Bioinformatics and Computational Biology and Global Computing Lab, University
of Delaware, Newark, DE, USA
High performance computing (HPC) resources and
workloads are undergoing tumultuous changes. HPC resources are growing more
diverse with the adoption of accelerators; HPC workloads have increased in
size by orders of magnitude. Despite these changes, when assigning workload
jobs to resources, HPC schedulers still rely on users to accurately
anticipate their applications’ resource usage and remain stuck with the
decades-old centralized scheduling model.
In this talk we will discuss these ongoing changes
and propose alternative models for HPC scheduling based on resource-awareness
and fully hierarchical models. A key role in our models’ evaluation is played
by an emulator of a real open-source, next-generation resource management
system. We will discuss the challenges of realistically mimicking the
system's scheduling behavior. Our evaluation shows
how our models improve scheduling scalability on a diverse set of synthetic
and real-world workloads.
This is joint work with Stephen Herbein
and Michael Wyatt at the University of
Delaware, and Dong H. Ahn, Todd Gamblin,
Don Lipari, Adam Moody, Tapasya Patki,
Bronis de Supinski ,
Thomas R.W. Scogland, Marc Stearman,
Jim Garlick, Mark Grondona,
Tamara Dahlgren, David Domyancic, and Becky Springmeyer at the Lawrence Livermore National
Laboratory.
Back to Session IV
|
Challenges in big data computing on HPC
platforms
Michela Taufer
Dept. of Computer and Information Sciences,
Biomedical Engineering
and Center for
Bioinformatics and Computational Biology and Global Computing Lab, University
of Delaware, Newark, DE, USA
Data analytics and data intensive workloads have
become an integral part of large-scale scientific workloads. Still efforts to
enable big data processing on high performance computing (HPC) platforms are
in their infancy and data intensive applications are not fully taking
advantage of the rapidly changing hardware and software technology landscape
in HPC.
In this talk, we explore trend and opportunities
when dealing with data intensive applications on the next generation HPC platforms.
Specifically, we tackle problems and propose solutions to schedule scientific
applications on increasingly bursty resources and
transform the centralized nature of data analysis into a distributed approach
that is performed in situ to
supports a broad range of molecular dynamics simulations. Our proposed
solutions go beyond HPC and develop opportunities for interdisciplinary
collaborations.
Back to Session VIII
|
Bootstrapping
an HPC Ecosystem
A Retrospective on Arm’s First Six Years in
High Performance Computing
Eric Van
Hensbergen
ARM Research, Austin, TX, USA
In late
2011, Arm’s participation in the Montblanc project launched its foray into
high performance computing as part of a larger strategy around expanding its
influence in the server market. A
little over six years later with ongoing projects in Europe, the US, and
Asia, the first large scale systems are being deployed based on Arm
technology with more to come in the coming months and years. This talk will cover some of the challenges
along the way, an overview performance of some of the now generally-available
platforms, and the future opportunities presented by recent additions to the
Arm architecture specifically to address the high performance computing and
data analytics market.
Bio
Eric Van Hensbergen is currently a Fellow at Arm working in the
research division out of the Austin,
TX design center.
He leads the software and large scale systems research group and is
senior director of Arm’s HPC effort. The group's activities include exploring
the place of ARM within high performance computing, data centers,
and investigating next generation concepts in operating systems, runtimes,
and systems software. Prior to Arm he
worked at IBM Research for 12 years and at Bell Laboratories for 5 years.
Back to Session II
|
How To Go Beyond the Limitations of the
Current Benchmarking Methodology?
Vladimir
Voevodin, Jack Dongarra
Moscow State University, Research Computing Center, Moscow, RUSSIA
The main disadvantage of the existing approach to
compare computer platforms based on Top500, Graph500 and HPCG is the choice
of too limited number of algorithms underlying the lists. In such a
situation, it is difficult to draw any conclusion about the performance of
computers on applications that rely on other algorithmic approaches. The AlgoWiki project is dedicated to describing the parallel
structure and key features of various algorithms from different areas. The
descriptions are intended to provide complete information about algorithm’s
properties, which are needed to adequately assess their implementation
efficiency for any computing platform. The algorithms underlying Linpack, Graph500 and HPCG, among others, are represented
in AlgoWiki and correspond to three points out of
the total multitude of algorithms in the project. Giving the computing
community an opportunity to submit and save the execution results for any
algorithm presented in AlgoWiki, we can
substantially improve comparing computing platforms and move from the three
points to an analysis based on dozens, if not hundreds of various algorithms.
We propose an approach to extend the existing methodologies to compare
various computing platforms using the wide and constantly growing algorithmic
potential of the AlgoWiki encyclopedia.
Back to Session III
|
Kakute: A Precise, Unified Information Flow
Analysis System for Big-data Security?
Amy Wang
The University of Hong Kong and Zhejiang University,
CHINA
Big-data frameworks (e.g., Spark) enable
computations on tremendous data records generated by third parties, causing
various security and reliability problems such as information leakage and
programming bugs. Existing systems for big-data security (e.g.,
Titian) track data transformations in a record
level, so they are imprecise and too coarse-grained for these problems.
Information Flow Tracking (IFT) is a conventional approach for precise
information control. However, extant IFT systems are neither efficient nor
complete for big-data frameworks, because theses frameworks are
data-intensive, and data flowing across hosts is often ignored by IFT.
This talk presents Kakute,
the first precise, fine-grained information flow analysis system for
big-data. Our insight on making IFT efficient is that most fields in a data
record often have the same IFT tags, and we present two new efficient
techniques called Reference Propagation and Tag Sharing. In addition, we
design an efficient, complete cross-host information flow propagation
approach. Kakute effectively detected 13 realworld security and reliability bugs in 4 diverse
problems, including information leakage, data provenance, programming and
performance bugs. This work got best paper award in ASSAC17.
Back to Session VIII
|
D-Wave's Approach to Quantum Computing: Past,
Present, and Future
Colin
Williams
D-WAVE System Inc., Strategy and Corporate
Development, USA
Quantum computing promises to revolutionize computer
technology as profoundly as the airplane revolutionized transportation. After
decades of incubation, early generation quantum computers are finally
appearing that allow people to begin experimentation in earnest. In this
talk, I will describe D-Wave's approach to quantum computing, explain its
pros and cons with respect to competing schemes, and give the rationale
behind our design choices. Furthermore, I will give examples of how the
native optimization and sampling capabilities of our quantum processor can be
exploited to tackle problems in a variety of fields including healthcare,
physics, finance, simulation, artificial intelligence, and machine learning.
BIO
Colin P. Williams is Vice President Strategy & Corporate
Development at D-Wave Systems Inc., reporting directly to the CEO. He has
spent over 20 years in quantum computing and has developed and patented
algorithms and applications for both gate model and annealing model
approaches. Prior to joining D-Wave, Colin was a Senior Research Scientist
(SRS) and Program Manager for Advanced Computing Paradigms at the NASA Jet
Propulsion Laboratory, California Institute of Technology. Earlier, as an
acting Associate Professor of Computer Science at Stanford University, he
devised, developed, and taught Stanford's first courses on quantum computing
& quantum communications, and computer-based mathematics. Colin earned
his Ph.D. in artificial intelligence from the University of Edinburgh in 1989
and wrote “Explorations in Quantum Computing,” one of the first textbooks in
the field.
Back to Session VI
|
Who [Should] Cares about HPC Software
Robert
Wisniewski
Exascale Computing, INTEL Corporation, New York, NY,
USA
In this talk I will discuss challenges facing the
future of HPC software. I will examine
them both from a technical perspective as well as an ecosystem
perspective. The observations will be focused
around the type of systems installed at supercomputer centers
around the world, but not necessarily limited to them. I will then describe the approach we are
taking at Intel to address some of the challenges and describe how OpenHPC is an important part of the equation.
Back to Session III
|
Scaling Deep Learning to Thousands of GPUs
Rio
Yokota
Global Scientific Information and Computing Center, Advanced Computing Research Division, Advanced
Applications of High-Performance Computing Group, Tokyo Institute of
Technology, Tokyo, JAPAN
ImageNet has become a common benchmark for large
scale distributed deep learning, where teams at Facebook, UC Berkeley,
Preferred Networks have independently performed runs on thousands of GPUs.
The current state-of-the-art can train ImageNet using ResNet-50 for 90 epochs
in about 15 minutes. However, data-parallel implementation of such large
scale deep learning requires very large batch sizes, which has a detrimental
effect on both the optimization and generalizability. We are currently
investigating alternative optimization methods that are less sensitive to the
increase in batch size. Large scale runs have been conducted on TSUBAME3.0
using 2048GPUs.
Back to Session V
|